Exploring Probability Through a Philosophical Lens: Part I
Written on
Foundations of Probability
This essay marks the beginning of a series that investigates the fundamental aspects of probability, focusing on various rationalizations. A subsequent essay will provide a critique of probability as a concept.
You can find the follow-up essay here:
The Nature of Empirical Knowledge
Empirical knowledge is generated from the observation of data points, followed by the construction of distributions—whether explicitly or implicitly formed. This raises the question of whether empirical knowledge is truly achievable without prior information guided by reason or a priori knowledge. Historical evidence suggests that humans often struggle to derive conclusions through abstract reasoning alone, indicating limited prospects for acquiring knowledge.
The Elusiveness of Probability Definitions
The definitions of probability are notoriously ambiguous. Mathematically, defining probability is relatively straightforward, revolving around the counting of 'equally likely' outcomes within a set. However, beyond the mathematical framework, the true essence of what constitutes probability remains unclear.
Understanding the nature of probabilities significantly influences reasoning. For instance, consider the fine-tuning argument for the existence of God. The assumption that "the constant is remarkably finely tuned" leading to the conclusion that "it is unlikely to occur without divine intervention" reveals the limitations of the mathematical definition of probability.
The implication of this argument can be analyzed through simple Bayesian probability. If we can establish a distribution that represents the likelihood of a deity's existence (and subsequently their role in creating life through finely-tuned constants), we can then compare this with the probability distribution of the physical constants' values if a deity does not exist. However, this approach leads to a fundamental dilemma: how can we create a probability distribution for a necessary being? Moreover, how could we even construct a probability distribution for physical constants?
The essence is that a probability distribution cannot encapsulate 'pure randomness' (whatever that may entail). If such a distribution existed, it would more accurately reflect uncertainty rather than alternative outcomes. In this context, there is no 'sample space' of potential outcomes.
The Challenge of Applying Probability
It may be that probability has limited applicability in this scenario, even though it feels unsatisfactory to disregard evidence concerning one of life’s greatest philosophical inquiries.
What about in the realm of Science? We might ponder the probability that, given an actual set of rules governing the universe, sufficient experimentation could lead to erroneous conclusions—eventually hitting a barrier that hampers further understanding. This scenario resembles navigating a landscape of mountains: consistently moving upwards ensures reaching the peak of one mountain, but not necessarily the highest one.
This 'gradient descent' method exemplifies the scientific process: observing, then adjusting theories to enhance compatibility with those observations. Is there a distribution of potential scientific theories we could potentially discover?
At the very least, this highlights the inadequacy of our current comprehension and formulation of probabilities. Just because 'things have worked out' does not exempt us from grappling with these challenging questions.
Probability in a Deterministic Framework
Consider a scenario where an individual selects an integer between 1 and n, and you attempt to guess their choice. What does it mean for that person to choose 'randomly'? With enough information, you could potentially predict the choice of a random number generator (or individual) accurately. In this situation, we utilize probabilities as a model for our limited understanding of mechanisms.
With ample observations, it appears that the cumulative effect of these unknown causes results in each number being selected with equal frequency. In a deterministic world, multiple possibilities do not exist. The probability distribution you adopt, assigning a probability of 1/n to each number, poorly predicts the singular true outcome, which occurs (1/n)^k times within the model, despite the inevitability of the deterministic nature of the world.
Imagine a character named Steve living on a two-dimensional plane. I methodically plot coordinates to form the graph of sin(x). However, Steve only sees a partial view of the graph and can never definitively conclude that it represents sin(x) rather than countless other polynomials that could pass through the same points. Fundamentally, Steve will remain uncertain unless he possesses prior knowledge about the kind of graph being drawn. He only observes finite data points—at any moment, an errant point could undermine his hypothesis that the line represents sin(x).
In a deterministic world, we embody Steve! Only by observing all data points can uncertainty be eliminated—but this is not feasible.
Can Steve make valid deductions about the graph beyond the points he can see?
In science, we essentially observe points plotted and extrapolate equations. Is predictive accuracy a sufficient test? What if Steve is predisposed to interpret the data as sin(x), while I am actually plotting a polynomial that closely resembles sin(x) within the observed range?
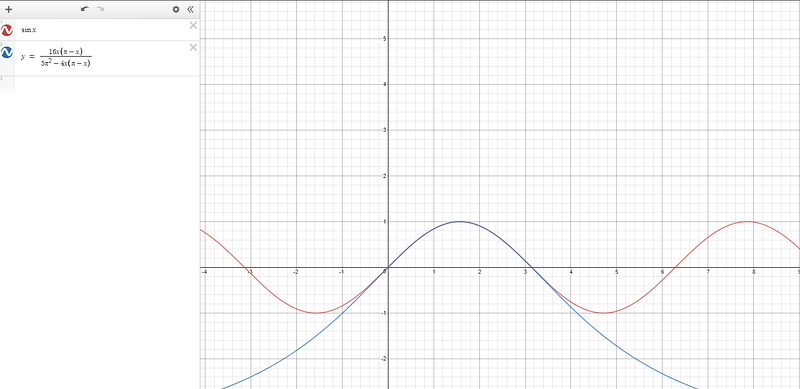
Probability in a Realm of True Randomness
In this context, multiple outcomes are indeed plausible. It is essential to recognize that the worlds of probability and determinism appear identical from our perspective. In the deterministic world, we cannot ascertain that each data point was not predetermined, just as in the probabilistic world, we cannot confirm the presence of genuine randomness.
In a world governed by randomness, if we were to exist within a deterministic framework, any set of data points could be consistent. What does this imply? Does the occurrence of a random event generate multiple worlds (and is it predetermined which world we inhabit)? This dilemma arises from the persistent reliance on the mathematical properties of random variables, which were initially intended to reflect real-world phenomena.
We are attributing numerical values to elements in the world, thus we should comprehend what these numbers signify.
If we rely on our vague understanding of random variables, we arrive at a crucial insight:
In mathematics, we typically begin with a distribution and derive outcomes. In contrast, real life entails observing and gradually constructing models of our surroundings. For example, an individual who has faced repeated mistreatment may develop a different perspective on trustworthiness compared to someone who has never experienced deception. This discrepancy might explain why children often exhibit greater trust.
When we only perceive data points without understanding the outcomes, we can never know the underlying distribution.
The Role of Priors in Statistical Inference
Ultimately, we confront the impossibility of attaining knowledge. We often evade this reality through an evolutionary inclination towards certain priors.
Consider the following scenario:
Two individuals witness an event without any prior acquaintance. I question one of them, who asserts that A occurred. My uncertainty lingers—could this person be lying? If I believe they have no substantial reason to deceive (effectively forming a distribution regarding their truthfulness), I may feel reassured.
Next, I interrogate the second individual, who also claims that A transpired. This testimony bolsters my conviction, as my mind has a prior distribution concerning the frequency of lies. Unless I have compelling evidence suggesting collusion between the two witnesses, it is reasonable to conclude that A is indeed the event that unfolded.
We only observe data points, and our interpretation of these points is contingent upon an underlying distribution. By accumulating numerous data points, we can formulate a distribution. However, creating a distribution necessitates considering the distribution of distributions. Our rationale for establishing a distribution fundamentally hinges on consistency—specifically, how well the model aligns with the data. Yet, our confidence in the model's correctness must also depend on the distribution of models!
In an illustrative example, if a computer generates a normal distribution with a mean of 0, with each x value denoting the mean of the normal distribution for a specific variable, then if your data exhibits a mean of 3, the most accurate estimate for the underlying distribution will lean slightly toward 0, as this is where the probability mass for the underlying distribution resides.
You may question the necessity of distributions concerning distributions. Nevertheless, in both random and deterministic contexts, distributions serve as our tool for quantifying uncertainty regarding a deterministic system or the probabilities inherent in a genuinely random environment. Thus, if you fail to quantify a distribution for the distributions, you remain uncertain about the uncertainty or randomness involved. Essentially, you have made no epistemological advancement.
This reasoning can be extended to the distribution of distributions. However, at this stage, we are attempting to conduct statistical inference based on a single data point. (The logic implies an infinite regression.)
Conclusions and Future Directions
In conclusion, our understanding of probabilities remains fundamentally flawed. This realization will set the stage for the second essay, which critiques the broader theory of probability.
Next up: