Establishing a Data-Driven Culture through Effective Engineering
Written on
Chapter 1: The Role of Data Engineers in Shaping Culture
In the quest for consistent and dependable data, one crucial step is to hire additional data engineers and establish a robust data observation framework.
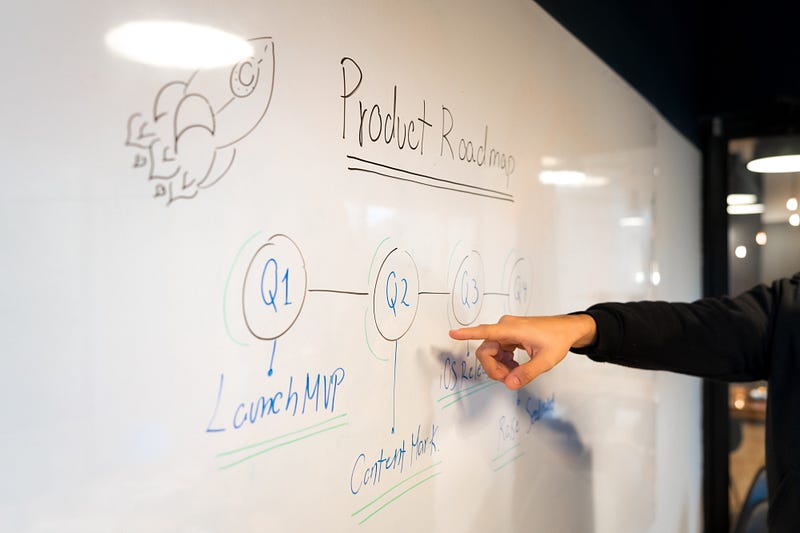
Photo by Slidebean on Unsplash
As I settled in to rectify yet another report on a Friday evening, I felt the weight of being the sole data engineer at a small startup. My role revolved around addressing data issues to support the organization effectively.
It's easy to overlook the fact that these reactive measures to fix reports are merely short-term fixes in our journey toward establishing a data-driven culture. Here, I aim to propose a more sustainable approach that benefits both the data engineers and the organization as a whole.
My journey began as a consultant collaborating with some of the leading companies in the industry. I was fortunate to be part of a team that prioritized analytics. This group shared a common passion for data, engaging in brainstorming sessions where ideas flowed freely, and constructive feedback was the norm.
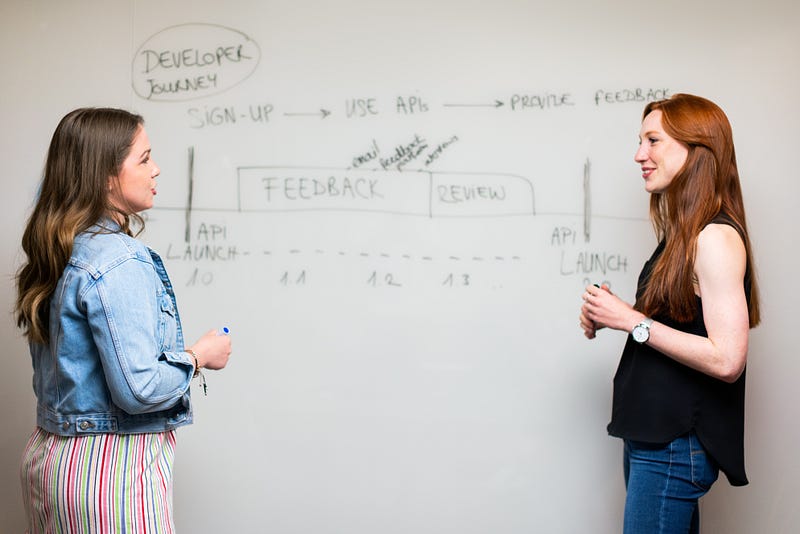
Photo by ThisisEngineering RAEng on Unsplash
While the boundaries of our projects were well-defined, the methodology for executing them was left to the teams. Our top priority was ensuring clean data quality and simplifying the code.
We had established processes in place, including daily stand-ups and code reviews. Engineers, scientists, and designers collaborated to identify tasks, and most importantly, we had the necessary resources to accomplish our objectives.
A critical resource I want to emphasize is the backing of leadership. They desire clean, reliable data to make informed decisions, but often overlook the significant behind-the-scenes efforts required from data engineers. There’s a common misconception that simply hiring a data engineer will resolve all data pipeline issues.
However, many existing pipelines are neither stable nor robust. They often exist in fragmented locations, such as an outdated Mac in the office, an underutilized virtual machine in the cloud, or a subpar Azure data factory pipeline lacking proper monitoring. It’s no wonder that upper management is bewildered when they receive feedback from both employees and customers about the persistent failures of these makeshift data solutions.

Photo by SEL?M ARDA ERYILMAZ on Unsplash
In a startup environment, this constant reactive problem-solving can be particularly daunting, especially when data teams often consist of fewer than five members and lack dedicated management. Typically, these teams include data analysts, data engineers, and data scientists.
The most significant challenge lies in securing the necessary resources—not just financial ones. Leaders must shift their focus toward a proactive strategy for data pipelines rather than draining their data teams with ongoing reports and inconsistencies. While addressing these issues is indeed necessary, establishing solid, reliable data pipelines with proper monitoring is paramount.
A proactive approach to data pipelines will only gain traction once a startup or any business evolves into a more data-centric environment. This evolution hinges on the realization that technology is not the primary obstacle; instead, cultural barriers and internal processes hinder an effective data strategy. According to a 2021 survey by NewVantage Partners, only 7.8% of 85 firms identified technology as the main hurdle, while 92.2% attributed challenges to cultural and procedural issues.¹ Therefore, dismantling these cultural barriers is essential for a successful data-driven startup. While acquiring the right technology or hiring additional personnel can be beneficial, these actions alone will not transform a data-resistant culture. As management consultant Peter Drucker famously stated, "Culture eats strategy for breakfast."
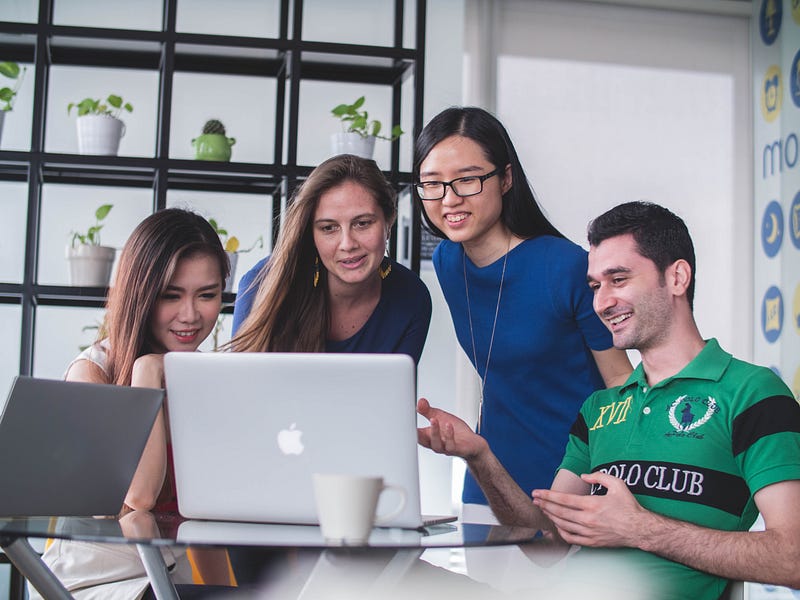
Photo by Mimi Thian on Unsplash
The focus should instead be on securing the buy-in and support of executives and upper management. In Thomas Davenport's book, "Analytics at Work: Smarter Decisions, Better Results," he highlights a case where a consumer goods company failed to reallocate its marketing budget to more effective channels due to a lack of trust in their own analysis.² This reluctance demonstrates how leadership can undermine the significance of internal data, resulting in demotivated employees who feel their contributions are undervalued.
To foster a closer relationship between business and analytics, David Waller suggests in his article, "10 Steps to Creating a Data-Driven Culture," that top managers must set the expectation that decisions should be data-driven.³ A practical approach to implement this is by presenting critical data to executives, encouraging them to engage with it daily. Executives should be empowered to ask insightful questions, gain context, and ultimately base their decisions on this data.
Before rolling out such initiatives, it's important to assess the current resources available. My colleagues and I have often been stretched thin, tasked with resolving issues or temporarily patching data pipelines to meet demands. Management often expresses surprise when reports that are visible to customers and employees fail to hold up consistently.
While fixing these reports is crucial, it’s only after establishing reliable data pipelines that we can consistently generate core reports, thereby earning the trust of both employees and customers. This aligns with Waller's ninth step in creating a data-driven culture, which emphasizes trading flexibility for consistency, at least in the short term.³
Selecting which data pipelines to prioritize is challenging. How do we determine the robustness of a data pipeline? One key criterion is data downtime, which can stem from partial, incomplete, inaccurate, or outdated information. If we evaluate our reports today, how reliable are they? Can we trust the data? The answer is often a resounding "No." We need deeper insights into these data pipelines before we can assess their priorities. The tools we use are secondary to the importance of gathering metrics, traces, and logs. I acknowledge that this process can be time-consuming or even daunting. Many tools we rely on to integrate data pipelines offer incomplete metrics and lack seamless integration with metric tools. I suggest focusing first on the most critical data pipeline that could cause significant issues if it fails.
To identify which data pipelines are most critical, we should define their service level objectives (SLOs) and service level indicators (SLIs). SLOs encompass factors like data freshness, correctness, and load balancing, while SLIs should meet established levels over specific time frames. For further information, Google provides definitions and insights on these terms in their articles. By determining which data pipelines hold the strictest criteria and impact both customers and employees, we can effectively prioritize them.
Once we have ranked the data pipelines, the next step is to allocate appropriate resources. Constructing robust pipelines becomes a more manageable challenge when we can hire or assign data engineers and software engineers dedicated to this task. Regardless of how many new hires we make, the key to rebuilding these pipelines lies in their simplicity and reliability.
With robust data pipelines and straightforward reports in place, we can address the inherent inaccuracies within these reports. Understanding the level of uncertainty behind collected data helps us identify gaps. This awareness paves the way for running experiments, enabling us to learn more about user interactions with our product. Brent Dykes’ article, "Creating A Data-Driven Culture: Why Leading By Example Is Essential," illustrates how former Google executive Marissa Mayer successfully tested 41 different shades of blue for Google’s advertising links, resulting in an increase of £200M in ad revenues that year.⁴ Thus, cultivating a data-driven culture can lead to revenue growth and deeper insights, necessitating the ongoing need for more data and, consequently, more data engineers.
Data engineers are fundamental to fostering a data-driven culture. They provide the clean, robust data pipelines necessary to encourage wider organizational data usage. Leadership must engage with these reports regularly to instill a data-first mentality throughout the company. While we may encounter uncertainties in the reported data, we embrace these as opportunities for experimentation and growth, rather than viewing them as setbacks.
The first video titled "Building a Winning Data Engineering Culture" delves into the elements essential for cultivating a successful culture among data engineers, highlighting best practices and strategic approaches.
The second video, "A Step-by-Step Guide to Building a Data-Driven Culture in Your Business Using Power BI," offers practical insights on how to leverage Power BI for fostering a data-centric environment in your organization.
References
[2] Davenport, Thomas H., et al. Analytics at Work: Smarter Decisions, Better Results. Harvard Business Review Press, 2010.